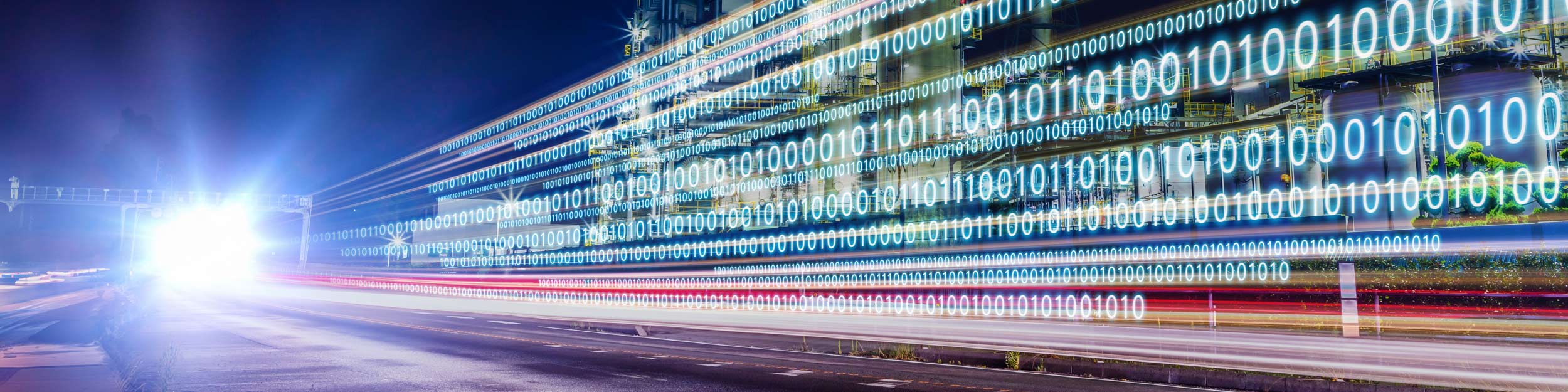
Digital Transformation
Management & Society
The research area "Digital Transformation" complements the study programs of Management, Communication & IT as well as Digital Business & Software Engineering respectively in the fields of applied research and development of practice-oriented solutions for digitalization and their comprehensive integration into practice. We work on technical, social, organisational and individual aspects in an interdisciplinary way.
In the context of digital transformation, the following aspects reflect our values and inspiration:
- The secure, analytical handling of data
Ensuring the ethical management and analysis of data, prioritizing privacy, security, and the responsible use of information to benefit society while minimizing environmental impact. - The interaction of people with technical systems
Promoting fair engagement between individuals and technical systems, ensuring accessibility, inclusivity, and the enhancement of human well-being. - The design of new work environments
Creating work environments that are ethically sound, focusing on the well-being of employees and our environment. - The adaptation of work and production processes in real as well as virtual worlds
Modifying work and production processes to be responsible and sustainable, integrating practices that reduce environmental impact and promote social equity in both physical and digital spaces.
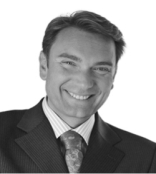
If you have any questions regarding this research area, please contact us:
digitaltransformation@mci.edu
Data Analytics & Business AI
Through Data Analytics & Business AI companies are empowered to make smarter decisions, streamline operations and drive innovation. By analyzing vast amounts of data, they are able to uncover hidden patterns and insights, enabling them to personalize customer experiences, optimize supply chains, and predict potential risks. This data-driven approach fosters efficiency, fuels growth, and ultimately enhances profitability. It is not just focusing on technological advancement but rather treats data as a strategic asset that transforms how companies operate and compete in the modern landscape.
- IoT Integration & Data Collection
- Data Visualization & Dashboards
- AI-powered Knowledge Management & Decision Support Tools
- IT System Architecture Enhancement
- Data Engineering, Pipeline Development & Data Lifecycle Management
IT Security & Privacy
IT security and privacy contribute to the secure handling of data and information systems, which becomes ever more relevant in the course of increasing digitalization. Specifically, we focus on the security of IoT devices and machine learning algorithms.
- IoT Security
- Security & Privacy of Machine Learning
- Automation of Security Assessment
- Encryption Technologies
- Multimedia Security
Digital Interaction & Human-Centered Software Engineering
Digital Interaction & Human-Centered Software Engineering explores the dynamic interplay between people and technology in both work and personal settings. The field focuses on (1) understanding user behavior across and in specific use domains (e.g., Acceptance of Chatbots in travel logistics) and (2) designing user-centered experiences that are intuitive, engaging, and accessible (e.g., Usability of a digital pen & paper application for the Elderly). Overall, our aim is to ensure that technological solutions are not only functional, but also meaningful, impactful, and useful for their users.
- Interaction Design & User Needs
- Engaging & Positive User Experiences
- Digital Behavior
- Technology Acceptance
- Human-centered Software Engineering & Development
Operational Excellence & Agile Governance
The improvement of business decisions and the establishment of stable processes in the company - considering rapidly changing technologies, frameworks and the regulatory environment - are essential design fields of operational excellence and agile governance.
- Internet of Things
- Smart Production
- Data Integration
- Audits
- Compliance & IT Governance of Agile Action
Digital Communication & Next Gen Work
Digital Communication & Next Gen Work explores the transformative impact of digital technologies on communication practices and workplace dynamics. We investigate how emerging technologies shape the future of work, enhance collaboration, and influence organizational structures and cultures.
- Digital Communication Dynamics
- Next-Generation Work Skills
- Technology-Driven Leadership
- Impact of AI on Workplace Roles
- Virtual Collaboration Tools
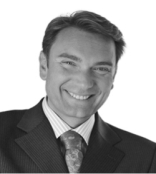
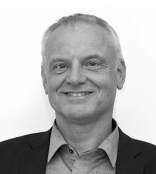
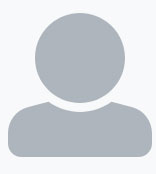
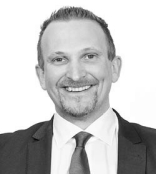
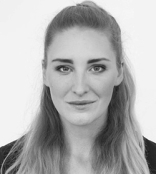
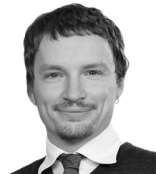
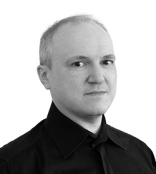
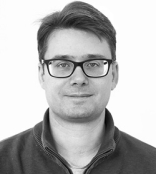
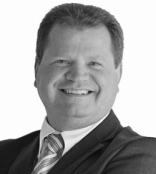
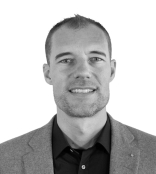
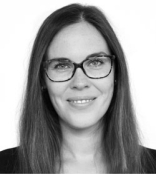
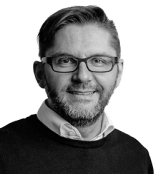
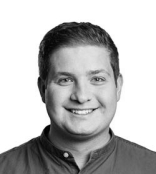
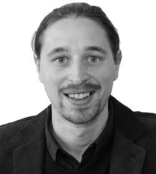
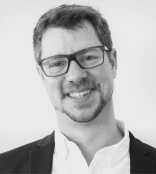
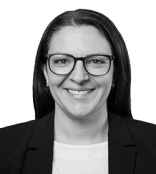
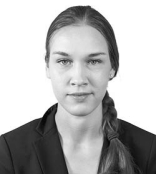
Entwicklung eines erweiterten Online-Simulationsspiels für Operational Excellence
2024 - 2025
PLG_RESEARCH_LEITER:
FH-Prof. Dr. Stephan Schlögl
FH-Prof. Dr. Reinhard Bernsteiner
Smart Inclusion - supporting the independence of people with disabilities using Smart Glasses
2022 - 2023
PLG_RESEARCH_LEITER:
Magdalena Posch, BA MA MA
PLG_RESEARCH_PRMITARBEITER:
Michael Freudenthaler, BA MA
Anna-Karina Sailer, BA MA
Franziska Scheele
AI4VET4AI - AI-powered Next Generation of VET
2023 - 2027
PLG_RESEARCH_LEITER:
FH-Prof. Dr. Stephan Schlögl
PLG_RESEARCH_PRMITARBEITER:
Annika Gnädinger, B.Sc. MA
FH-Prof. Dr. Reinhard Bernsteiner
FH-Prof. Dr. Christian Ploder
FH-Prof. Dr. Peter J. Mirski
https://www.ai4vet4ai.eu/
Prototypical Implementation of an intelligent tutoring system for introductory programming courses
2022 - 2024
PLG_RESEARCH_LEITER:
Assoz. FH-Prof. Matthias Janetschek, PhD
PLG_RESEARCH_PRMITARBEITER:
FH-Prof. Dr. Stephan Schlögl
FH-Prof. Dr. Christian Ploder
Secure Machine Learning Applications with Homomorphically Encrypted Data
2021 - 2024
PLG_RESEARCH_LEITER:
FH-Prof. Dr. Pascal Schöttle
PLG_RESEARCH_PRMITARBEITER:
Martin Nocker, MSc
Carina Kollmitzer
Advancing careers in mathematics and computer science Heidelberg Laureate Forum Foundation Project 2022+ in cooperation with MCI
2023 - 2025
PLG_RESEARCH_LEITER:
FH-Prof. Dr. Peter J. Mirski
PLG_RESEARCH_PRMITARBEITER:
Susann Kruschel, MSc
FH-Prof. Dr. Dietmar Kilian
Valentina Huter, BA MA
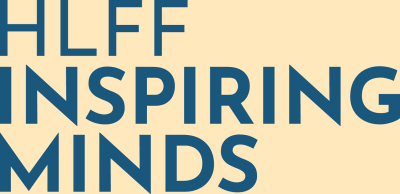
Smart Technology Monitoring II
2023 - 2024
PLG_RESEARCH_LEITER:
FH-Prof. Dr. Stephan Schlögl
PLG_RESEARCH_PRMITARBEITER:
Roman Blaas, BSc
Assoz. FH-Prof. Matthias Janetschek, PhD
FH-Prof. Dr. Oliver Som
Smart Villages / Regionalmanagement Wipptal
2020 - 2024
PLG_RESEARCH_LEITER:
FH-Prof. Dr. Christian Ploder
PLG_RESEARCH_PRMITARBEITER:
Lukas Heschl, BA MA
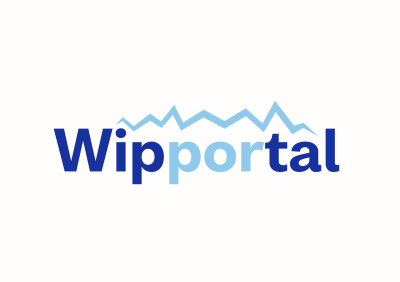
Ready, Immerse, Go!
2023 - 2026
PLG_RESEARCH_LEITER:
FH-Prof. Dr. Peter J. Mirski
PLG_RESEARCH_PRMITARBEITER:
FH-Prof. Aleksander Groth, PhD
CRYSTAL - Conversational Systems for Emotional Support and Customer Assistance
2024 - 2028
PLG_RESEARCH_LEITER:
FH-Prof. Dr. Stephan Schlögl
Smartify TT
2022 - 2024
PLG_RESEARCH_LEITER:
FH-Prof. Dr. Stephan Schlögl
PLG_RESEARCH_PRMITARBEITER:
Assoz. FH-Prof. Andrea Corradini, PhD
Assoz. FH-Prof. Matthias Janetschek, PhD
Game Over Eva(sion): Sicheres Deep Learning mit Spieltheorie
2019 - 2023
PLG_RESEARCH_LEITER:
FH-Prof. Dr. Pascal Schöttle
PLG_RESEARCH_PRMITARBEITER:
Florian Merkle, BSc MA
- Ploder, C., Allegro, A., & Bernsteiner, R. (2025). Quality Control and Management Systems for Lithium-Ion Battery Production: A Systematic Literature Review. Advanced Energy Conversion Materials, 6(1), 122-136. https://doi.org/10.37256/aecm.6120256547
- Längle, S. T., Schlögl, S., Ecker, A., van Kooten, W. S. M. T., & Spieß, T. (2024). Nonbinary Voices for Digital Assistants—An Investigation of User Perceptions and Gender Stereotypes. Robotics, 13(8). https://doi.org/10.3390/robotics13080111
- Hilbrich, M., & De Mecquenem, N. (2025). Microservices, a Definition Analyzed by ßMACH. Computer Science, 25(4). https://doi.org/10.7494/csci.2024.25.4.5423
- Alamäki, A., Khan, U. A., Kauttonen, J., & Schlögl, S. (2024). An Experiment of AI-Based Assessment: Perspectives of Learning Preferences, Benefits, Intention, Technology Affinity, and Trust. Education Sciences, 14(12). https://doi.org/10.3390/educsci14121386
- Merkle, F., Samsinger, M., Schöttle, P., & Pevny, T. (2024). On the Economics of Adversarial Machine Learning. IEEE Transactions on Information Forensics and Security, 1–1. https://doi.org/10.1109/TIFS.2024.3379829
- Schweitzer, R., Schlögl, S., & Schweitzer, M. (2024). Technology-Supported Behavior Change—Applying Design Thinking to mHealth Application Development. European Journal of Investigation in Health, Psychology and Education, 14(3), 584–608. https://doi.org/10.3390/ejihpe14030039
- Schadelbauer, L., Schlögl, S., & Groth, A. (2023). Linking Personality and Trust in Intelligent Virtual Assistants. Multimodal Technologies and Interaction, 7(6). https://doi.org/10.3390/mti7060054
- Höller, S., Dilger, T., Spiess, T., Ploder, C., & Bernsteiner, R. (2024). Awareness of Unethical Artificial Intelligence and its Mitigation Measures. European Journal of Interdisciplinary Studies, 15(2), 67–89. doi: https://doi.org/10.24818/ejis.2023.17
- Sternat, T., Bernsteiner, R., Ploder, C., & Dilger, T. (2024). Blockchains in health information systems: A literature review on use cases and status of implementation of blockchains for electronic health records. Human Systems Management, Preprint(Preprint), 1–18. https://doi.org/10.3233/HSM-230148
- Merkle, F., Weber, D., Schöttle, P., Schlögl, S., & Nocker, M. (2025). Less is More: The Influence of Pruning on the Explainability of CNNs. IEEE Access, 13, 87909–87927. https://doi.org/10.1109/ACCESS.2025.3569575
- Schlögl, S. (2025, May 7). Investigating the Usability of a Digitized Trail Making Test with Older Adults [Conference Presentation]. FFH2025 18. Forschungsforum der österreichischen Fachhochschulen, Vienna, Austria.
- Groth, A. (2024, July 4). The emergence of a firestorm: Following communicative chain reactions on Reddit [Conference presentation]. EGOS 2024, Milan, Italy.
- Groth, A. (2024, July 5). When the frustrations of many turn into a firestorm: The communicative constitution of a collectively forceful voice [Conference presentation]. EGOS 2024, Milan, Italy.
- Schöttle, P. (2025, February 20). The Cyber Resilience Act and its Meaning for Cybersecurity Vendors [Invited Talk]. AV-Comparatives Security Summit & Awards, Innsbruck, Austria.
- Mirski, P. (2024, December 9). KI in der Produktion [Invited talk]. BMW, Munich, Germany.
- Bernsteiner R. (2024, December 18). Praxisvortrag: Augmented Reality (AR), Internet of Things (IoT), Digitaler Zwilling (Digital Twin) [Invited talk]. DigiPro II: Digitalisierung@HTL, St. Pölten, Austria.
- Schöttle, P. (2024, December 17). Praxisvortrag: IoT-Sicherheit [Invited talk]. DigiPro II: Digitalisierung@HTL, St. Pölten, Austria.
- Schlögl, S. (2024, October 25). Acceptance, Trust, Personality & Gender - Investigating Inherent Challenges of Human-AI Interaction [Invited talk]. Ulysseus Innovationhub for Applied AI for Business & Education, Helsinki, Finland.
- Dilger, T. (2024, September 11). Praxisvortrag: Cybersicherheit und Datenschutz [Invited talk]. Kiwanislub, Kitzbühel, Austria.
- Dilger, T. (2024, May 17). Introduction of AI in Sales via Tableau CRM: Integrating AI into the B2B Sales Process [Conferences presentation]. EBEEC 2023, Vilnius, Lithuania.
News
Digital Transformation
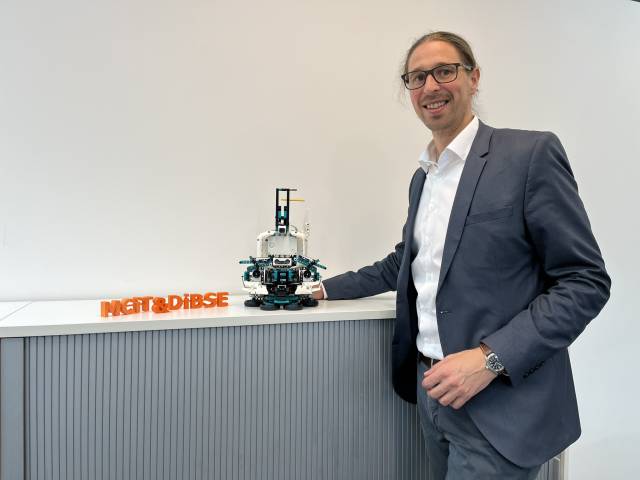
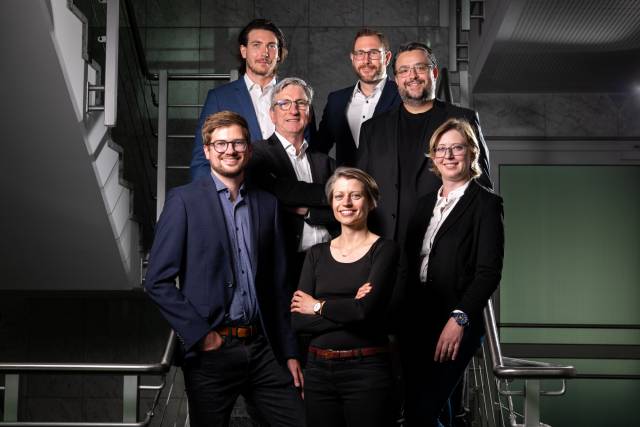
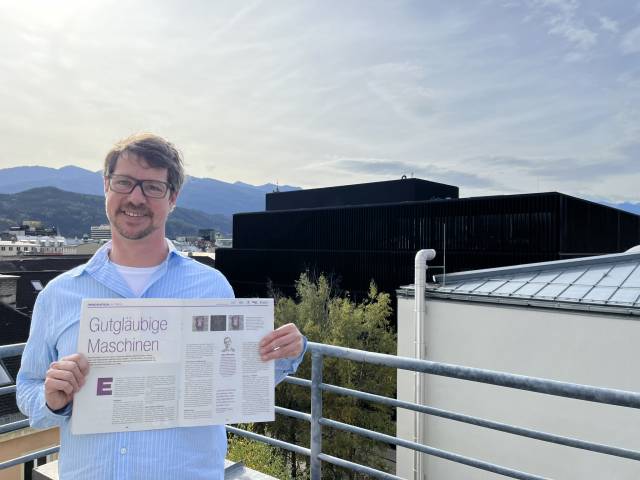